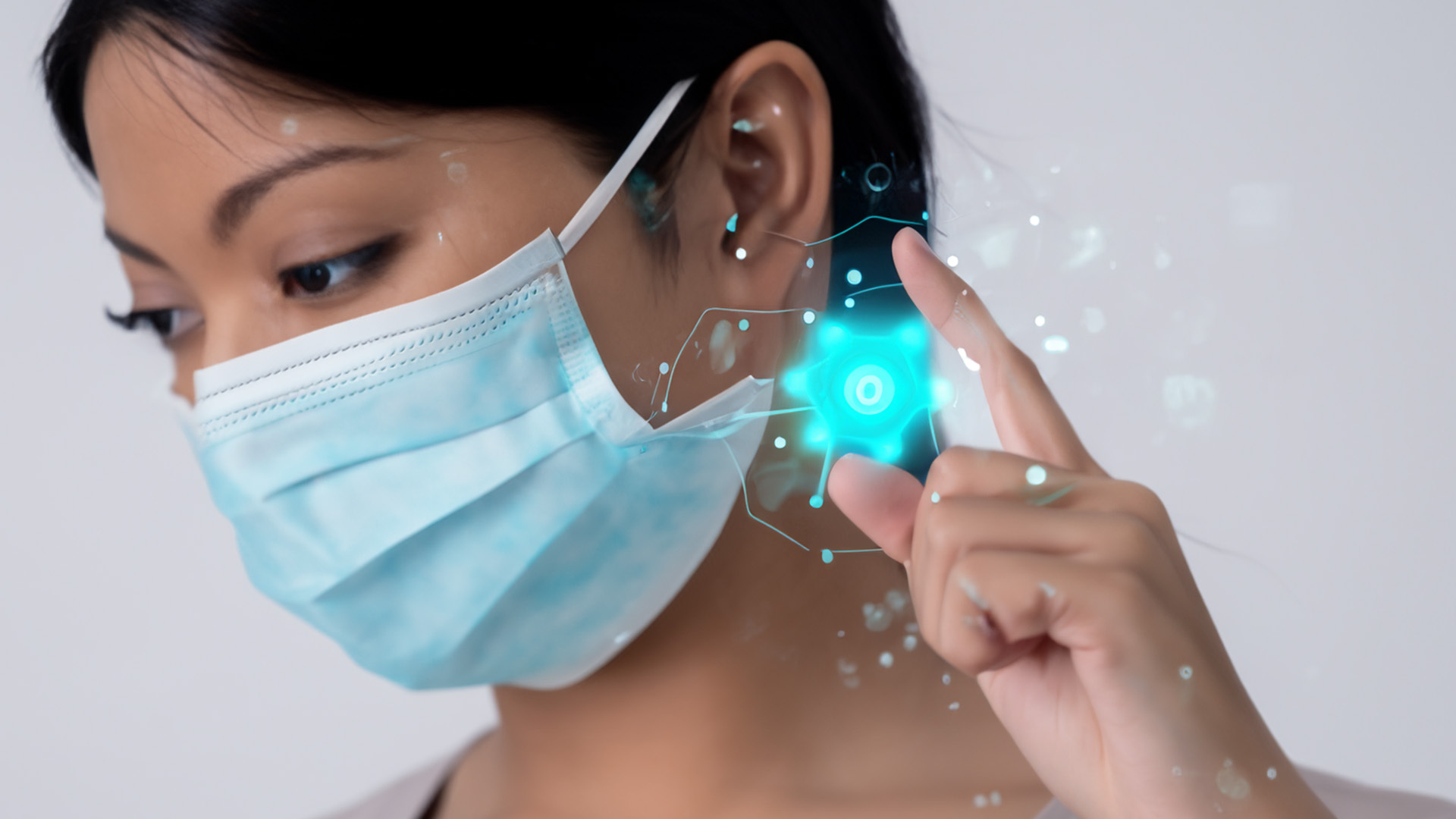
AI systems improve breast cancer detection rates
September 2023
By Wayne Kuznar for oncoXchange
Combining artificial intelligence (AI) diagnostic systems leads to improved risk assessment for breast cancers.
Use of a commercially available diagnostic AI system for lesion detection together with a mammographic texture model on a screening cohort of more than 119,000 Danish women, resulted in better assessment for both interval and long-term cancer detection than either texture risk or the AI system’s examination score. The findings were published in Radiology (2023;308:e230227).
In a statement issued by the Radiological Society of North America, lead study author Andreas D. Lauritzen, PhD, from the Department of Computer Science at the University of Copenhagen, Denmark, said, “In recent years and with the rise of power AI systems, research has been focused on using this technology to automatically detect breast cancers and to improve survival. However, such systems may also be used to measure the risk of a future breast cancer. In our study, we employed two AI systems: one for detecting cancer in suspicious lesions and another one for assessing risk by analyzing breast tissue patterns.
Diagnostic AI models trained to detect suspicious lesions on mammograms are well suited to estimate short-term breast cancer risk, and texture AI models, capable of identifying breast density, are better suited to assess long-term breast cancer risk, he explained.
For their study, the researchers used the diagnostic AI tool Transpara and a texture model they developed, trained separately and then combined, using examination data from 39,345 Dutch women. The combined model was tested on an independent Danish screening cohort of 119,650 Danish women. The average age of the women was 59 years.
The combination model improved risk segregation for women with a negative screening mammogram and at least 5 years of follow-up compared with both the AI system (area under the receiver operating characteristic curve [AUC], 0.73 vs 0.70; P < 0.001) and the texture model (AUC, 0.73 vs 0.66; P <0 .001).
Women identified by the combined model as having the 10% highest combined risk accounted for 44.1% (141 of 320) of interval cancers and 33.7% (472 of 1401) of long-term cancers, noted the investigators.
At 90% specificity, the combination model predicted future cancers with a sensitivity of 36.5%
A combination model that also included age and percentage mammographic density yielded an AUC of 0.72, which was not different from the combination model without these patient characteristics (AUC, 0.73; P = 0.24).
Using AI to identify breast cancer risk from a single mammogram will not only result in earlier cancer detection but can also improve the strain on health care systems due to the worldwide shortage of specialized breast radiologists, said Prof. Lauritzen.
“Essentially, women who attend mammography screening could now have their 5-year breast cancer risk measured instantaneously and without introducing unnecessary overhead in the screening clinics. And with supplemental imaging, like for instance, MRI [magnetic resonance imaging], for women at high risk, up to 36% of breast cancers could possibly be detected earlier according to our study,” he said.
A separate study that appears in The Lancet Oncology (2023;24:936-944) adds support for the use of AI in breast cancer detection. In this study, researchers analyzed scans from more than 80,000 women in Sweden who underwent a mammogram between April 2021 and July 2022. Half of the women were assigned to a group in which AI read the mammogram before it was analyzed by a radiologist. The other group had their mammograms read by two radiologists without the use of AI.
The group whose scans were read by a radiologist who also used AI had 20% more cancers detected than the group whose mammograms were read by two radiologists without the additional use of AI.
The screenings supported by AI resulted in a cancer detection rate of 6.1 per 1,000 screened women, compared with 5.1 per 1,000 in the control group. Further, the use of AI did not increase the number of false positives. In the intervention group, 75% of the 244 cancers detected were invasive and 25% of were in situ; in the control group, 81% of 203 cancers were invasive and 19% were in situ.
As alluded to by Prof. Lauritzen, an additional benefit of AI in this study was a reduction in reading workload of 44.3%. The researchers calculated that if radiologists read about 50 mammograms per hour, a single radiologist would spend 4 to 6 months less to read about 40,000 screening examinations with the help of AI than it would take two radiologists alone.
Comments (0)